1 year ago
#100505
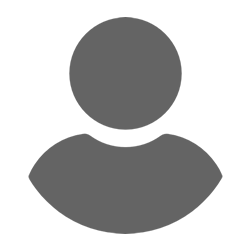
user17796629
Saving and loading Keras model with ELMO embedding layer
I'm training a Keras model for token classification with an ELMO layer. I will need to save the model for future use, I've tried with model.save_weights("model_weights.h5"), but then if I load them into a new model that I build, and then I call model.predict(...), I get results as if the model has never been trained. It looks like the configurations are not saved properly.
I am new with keras and tensorflow 1, and I'm not sure if this is the way to do it. Any help is welcome! I'm obviously missing something here, but I couldn't find sufficient on saving models with an elmo layer.
I am defining the model like this :
def ElmoEmbedding(x):
return elmo_model(inputs={"tokens": tf.squeeze(tf.cast(x, tf.string)),
"sequence_len": tf.constant(batch_size*[max_len])},
signature="tokens",
as_dict=True)["elmo"]
def build_model(max_len, n_words, n_tags):
word_input_layer = Input(shape=(max_len, 40, ))
elmo_input_layer = Input(shape=(max_len,), dtype=tf.string)
word_output_layer = Dense(n_tags, activation = 'softmax')(word_input_layer)
elmo_output_layer = Lambda(ElmoEmbedding, output_shape=(1, 1024))(elmo_input_layer)
output_layer = Concatenate()([word_output_layer, elmo_output_layer])
output_layer = BatchNormalization()(output_layer)
output_layer = Bidirectional(LSTM(units=512, return_sequences=True, recurrent_dropout=0.2, dropout=0.2))(output_layer)
output_layer = TimeDistributed(Dense(n_tags, activation='softmax'))(output_layer)
model = Model([elmo_input_layer, word_input_layer], output_layer)
return model
And I then I run the training like:
tf.disable_eager_execution()
elmo_model = hub.Module("https://tfhub.dev/google/elmo/3", trainable=False)
sess = tf.Session()
K.set_session(sess)
sess.run([tf.global_variables_initializer(), tf.tables_initializer()])
model = build_model(max_len, n_words, n_tags)
model.compile(optimizer="adam", loss="sparse_categorical_crossentropy", metrics=["accuracy"])
history = model.fit([np.array(X1_train), np.array(X2_train).reshape((len(X2_train), max_len, 40))],
y_train,
validation_data=([np.array(X1_valid), np.array(X2_valid).reshape((len(X2_valid), max_len, 40))], y_valid),
batch_size=batch_size, epochs=5, verbose=1)
model.save_weights("model_weights.h5")
If I try to load the weights in another session like the following, I get zero accuracy:
tf.disable_eager_execution()
elmo_model = hub.Module("https://tfhub.dev/google/elmo/3", trainable=False)
sess = tf.Session()
K.set_session(sess)
sess.run([tf.global_variables_initializer(), tf.tables_initializer()])
model = build_model(max_len, n_words, n_tags)
model.load_weights("model_weights.h5")
y_pred = model.predict([X1_test, np.array(X2_test).reshape((len(X2_test), max_len, 40))])
python
tensorflow
keras
elmo
0 Answers
Your Answer