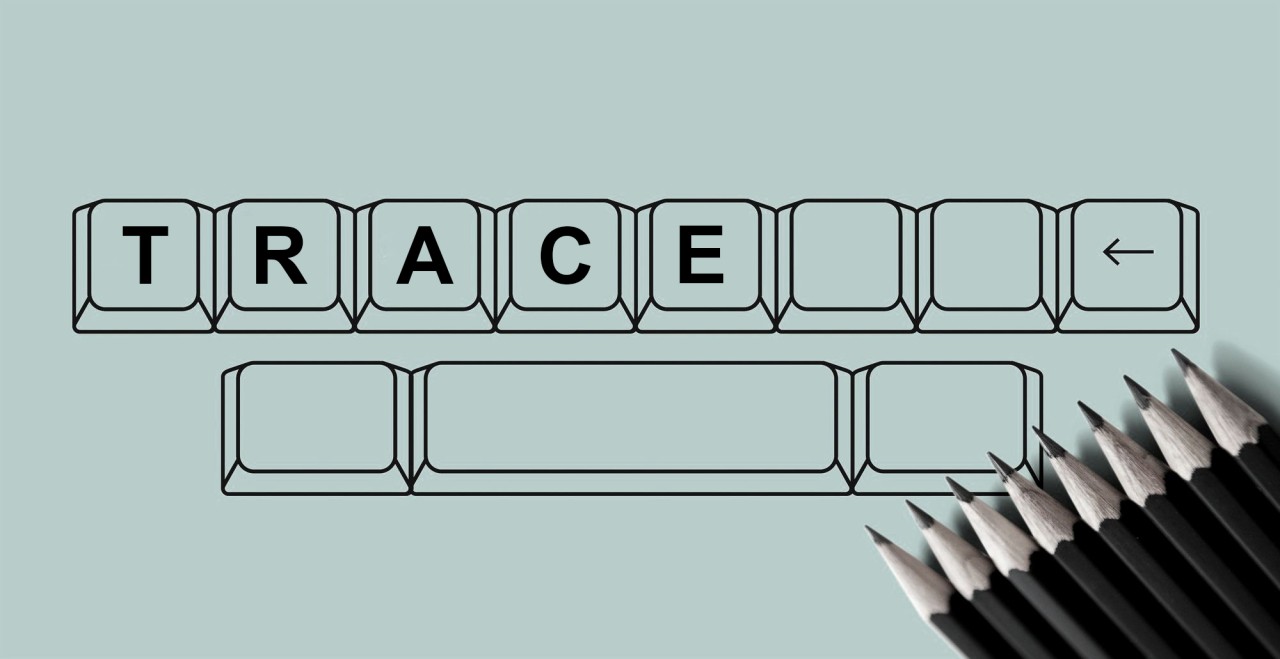
February 16, 2024
The TRACE framework
Introduction
The TRACE framework, standing for "Trustworthy Autonomy in Cognitive and Physical Environments," is a comprehensive approach developed to ensure the safety, reliability, and trustworthiness of autonomous systems, particularly in complex and dynamic environments. It integrates a variety of methods and techniques from different disciplines, including artificial intelligence (AI), robotics, control theory, and human factors, to address the challenges associated with deploying autonomous systems in real-world scenarios.
Key Components of the TRACE Framework:
Trustworthiness: Trust is a critical aspect of autonomous systems, especially when they operate in environments where human lives or valuable assets are at stake. The TRACE framework emphasizes the importance of building trust in autonomous systems through transparent decision-making, robust performance, and clear communication of system capabilities and limitations.
Autonomy: Autonomous systems are capable of making decisions and taking actions without direct human intervention. The TRACE framework focuses on enabling autonomy while ensuring that the system operates within predefined boundaries and adheres to established safety and ethical norms.
Cognition: Cognitive capabilities allow autonomous systems to perceive, reason, and adapt to changing environments and tasks. The TRACE framework incorporates cognitive modeling techniques to enhance the intelligence and flexibility of autonomous systems, enabling them to learn from experience and make informed decisions in complex and uncertain situations.
Physical Environments: Autonomous systems interact with physical environments, which may pose various challenges such as obstacles, dynamic conditions, and unpredictable events. The TRACE framework considers the physical aspects of the environment and develops techniques for sensing, perception, and navigation to ensure safe and efficient operation of autonomous systems in diverse settings.
Applications of the TRACE Framework:
The TRACE framework has broad applications across several domains, including:
Robotics: In robotics, the TRACE framework can be used to develop autonomous robots for tasks such as search and rescue, environmental monitoring, and industrial automation. By ensuring the trustworthiness of robotic systems, the framework enhances their reliability and safety in real-world applications.
Autonomous Vehicles: Autonomous vehicles rely on complex AI algorithms to navigate roads safely and efficiently. The TRACE framework provides a systematic approach to designing and testing autonomous vehicle systems, addressing challenges related to perception, decision-making, and interaction with human drivers and pedestrians.
Healthcare: In healthcare, autonomous systems are used for tasks such as medical diagnosis, patient monitoring, and drug delivery. The TRACE framework ensures that these systems operate reliably and ethically, maintaining patient safety and privacy while providing accurate and timely assistance to healthcare professionals.
Defense and Security: Autonomous systems play a crucial role in defense and security applications, including surveillance, reconnaissance, and border patrol. The TRACE framework helps ensure that autonomous defense systems operate with high levels of trustworthiness and integrity, minimizing the risk of unintended consequences or misuse.
Conclusion:
The TRACE framework represents a holistic approach to developing and deploying autonomous systems in a wide range of applications. By integrating principles of trustworthiness, autonomy, cognition, and consideration of physical environments, the framework provides a systematic methodology for ensuring the safety, reliability, and effectiveness of autonomous systems in complex and dynamic real-world settings. As autonomous technologies continue to advance, the TRACE framework will play a vital role in shaping the future of autonomous systems across various domains.
490 views